P-value Method Formula
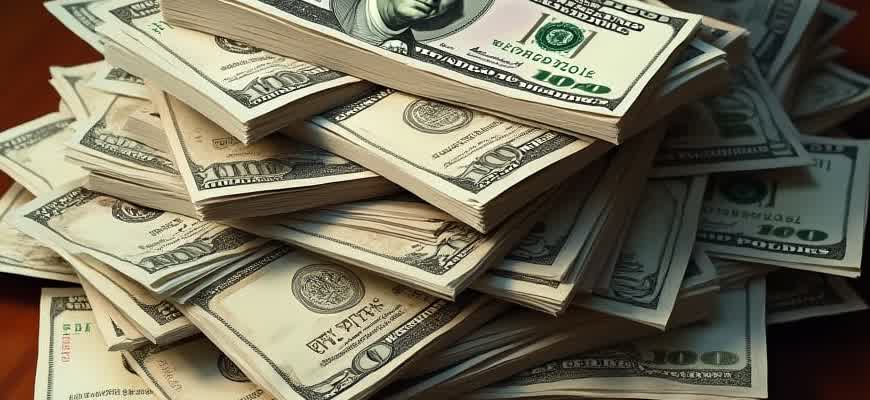
The P-value is a crucial concept in statistical hypothesis testing, helping researchers determine the strength of evidence against the null hypothesis. The smaller the P-value, the stronger the evidence against it. It is often used to assess the statistical significance of the results obtained in an experiment or study.
The formula for calculating the P-value depends on the type of test being performed, such as a one-tailed or two-tailed test. It involves comparing the test statistic to a distribution, typically the normal distribution or t-distribution, to assess the likelihood of obtaining a result as extreme as the one observed, assuming the null hypothesis is true.
Key Information: A low P-value (typically less than 0.05) suggests that the null hypothesis can be rejected, while a high P-value indicates insufficient evidence to do so.
- Step 1: Define the null and alternative hypotheses.
- Step 2: Choose the appropriate statistical test (e.g., t-test, z-test).
- Step 3: Compute the test statistic.
- Step 4: Find the corresponding P-value using the chosen distribution.
- Step 5: Compare the P-value with the significance level (α), usually 0.05.
Significance Level (α) | Action |
---|---|
α ≤ 0.05 | Reject the null hypothesis |
α > 0.05 | Fail to reject the null hypothesis |
Interpreting the P-value: What Does It Really Mean?
The P-value is a critical component in hypothesis testing, used to help determine the statistical significance of the observed data. However, interpreting it correctly is essential to avoid common pitfalls. Essentially, the P-value quantifies the probability of obtaining an effect at least as extreme as the one observed, given that the null hypothesis is true. It is important to remember that a smaller P-value indicates stronger evidence against the null hypothesis, but it does not directly prove the alternative hypothesis.
Understanding what the P-value represents can often be challenging. It is not a definitive measure of the truth of the hypothesis but rather a tool for assessing evidence. Misinterpretations can arise if one equates a low P-value with "proof" of a hypothesis. A deeper look into its significance helps clarify its limitations and proper usage in the context of statistical testing.
The P-value does not measure the probability that either hypothesis is true. It measures the probability of the observed data given the null hypothesis.
Key Concepts for Understanding the P-value
- Threshold for significance: A commonly used threshold is 0.05. If the P-value is less than this value, the result is typically considered statistically significant, meaning that the null hypothesis can be rejected.
- Large P-value: A P-value greater than 0.05 suggests weak evidence against the null hypothesis, leading to the conclusion that there is insufficient evidence to reject it.
- Small P-value: A P-value less than or equal to 0.05 indicates strong evidence against the null hypothesis, leading to its rejection in favor of the alternative hypothesis.
Interpreting the P-value Correctly
- Context matters: The P-value should be interpreted in the context of the study's design, sample size, and the underlying assumptions of the statistical test.
- Statistical significance does not imply practical significance: A small P-value indicates statistical significance, but this does not necessarily mean the effect size is large enough to be practically important.
- Consider the power of the test: A high P-value may not always mean that there is no effect. It could also suggest that the test lacks sufficient power to detect an effect, especially in cases of small sample sizes.
Example of P-value Interpretation
P-value | Interpretation |
---|---|
< 0.05 | Strong evidence against the null hypothesis. It is typically rejected. |
0.05 – 0.10 | Weak evidence against the null hypothesis. It may not be rejected, but results are marginally significant. |
> 0.10 | Weak evidence against the null hypothesis. It is typically not rejected. |
Common Mistakes in P-value Calculation and How to Avoid Them
When calculating the p-value for hypothesis testing, researchers often make errors that can lead to inaccurate conclusions. These mistakes can stem from misunderstandings of statistical concepts, incorrect application of formulas, or misinterpretation of results. It is important to recognize and correct these errors to ensure valid and reliable outcomes in statistical analyses.
Below are some of the most common mistakes encountered during p-value calculation and methods to prevent them. By addressing these issues, you can improve the accuracy of your statistical testing process and make more informed decisions based on the data.
1. Misinterpreting the P-value
The p-value is often misunderstood as the probability that the null hypothesis is true. In reality, it represents the probability of obtaining a result at least as extreme as the one observed, given that the null hypothesis is true. Misinterpreting this can lead to incorrect conclusions about the significance of the results.
Key Takeaway: A p-value of 0.05 means there is a 5% chance of observing the data (or something more extreme) under the null hypothesis, not that the null hypothesis has a 5% chance of being true.
2. Not Correcting for Multiple Comparisons
When conducting multiple hypothesis tests simultaneously, the chance of encountering a false positive increases. Without adjusting for this, the likelihood of rejecting a true null hypothesis increases. Common methods to control this risk include the Bonferroni correction or the False Discovery Rate (FDR) approach.
- Bonferroni Correction: Divide the significance level (α) by the number of tests to get a more stringent threshold.
- FDR Approach: This method adjusts the p-value threshold based on the proportion of false discoveries you are willing to tolerate.
3. Using the Wrong Test for the Data Type
Using the wrong statistical test can result in incorrect p-value calculations. For example, applying a t-test to non-normally distributed data can lead to invalid conclusions. Always ensure the test you choose is appropriate for the data type and assumptions.
- Check for normality if using parametric tests (e.g., t-tests).
- Consider non-parametric tests (e.g., Mann-Whitney U test) if data does not meet assumptions of normality.
4. Overlooking the Effect Size
While the p-value indicates statistical significance, it does not measure the strength or practical importance of an effect. Relying solely on the p-value can lead to ignoring the actual magnitude of an effect, which is critical in real-world decision-making.
Important: Always report the effect size along with the p-value to provide a more complete understanding of the results.
5. Rounding the P-value
Rounding p-values to a specific number of decimal places can lead to misleading interpretations. For instance, rounding a p-value of 0.049 to 0.05 may cause it to appear as non-significant when it is actually significant.
Unrounded P-value | Rounded P-value | Interpretation |
---|---|---|
0.049 | 0.05 | Non-significant (misleading) |
0.051 | 0.05 | Significant (misleading) |
When to Apply the P-value Approach in Statistical Data Analysis
In statistical analysis, choosing the right method for hypothesis testing is crucial to obtaining reliable results. The P-value method is widely used when assessing the strength of evidence against a null hypothesis. This approach helps determine whether the observed data significantly contradicts the null hypothesis or whether the results could have occurred by random chance. The P-value represents the probability of obtaining results at least as extreme as those observed, assuming the null hypothesis is true.
It is essential to apply the P-value method in situations where hypothesis testing is required, and when the assumptions for using the method are met. For example, the P-value method is often applied in fields such as medical research, market analysis, and experimental design, where researchers need to make data-driven conclusions. Below, we highlight when the use of the P-value method is most appropriate.
Key Situations to Use the P-value Method
- Testing a specific hypothesis: The P-value is useful when you need to determine whether a hypothesis about a population parameter (such as the mean or proportion) is supported by the data.
- Large sample sizes: The P-value method performs well with large datasets where the central limit theorem applies, making the data more likely to be normally distributed.
- Comparison of groups: When comparing two or more groups, the P-value can help determine whether observed differences are statistically significant.
Steps for Implementing the P-value Method
- State the null and alternative hypotheses: Define what you are testing (e.g., whether the population mean is equal to a specific value).
- Choose a significance level: Set a threshold (e.g., 0.05) to determine whether the results are statistically significant.
- Calculate the test statistic and P-value: Perform the appropriate statistical test (e.g., t-test or z-test) to obtain the P-value.
- Compare the P-value to the significance level: If the P-value is less than the chosen significance level, reject the null hypothesis.
Important Considerations
It is critical to remember that a P-value alone does not provide definitive proof of a hypothesis. A small P-value indicates strong evidence against the null hypothesis, but it does not confirm the alternative hypothesis. Additionally, P-values can be affected by sample size, and they should be interpreted in the context of the research design.
Common Scenarios for P-value Usage
Scenario | Test Type | Example |
---|---|---|
Medical Trials | T-test | Testing whether a new drug reduces symptoms compared to a placebo. |
Market Research | Chi-square test | Examining if customer satisfaction differs by region. |
Social Sciences | ANOVA | Determining if different teaching methods impact student performance. |
How to Select the Optimal Significance Level (Alpha) for Your P-value
When performing statistical tests, selecting the right significance threshold (denoted as alpha) is crucial to making accurate decisions. The significance level is the probability of rejecting the null hypothesis when it is actually true, i.e., making a Type I error. The common practice is to set alpha at 0.05, but this value is not always appropriate for every situation. Understanding how to choose an optimal significance level depends on the context of the test, the consequences of errors, and the nature of the data.
Choosing the right alpha involves considering the trade-off between Type I and Type II errors. A lower alpha reduces the likelihood of a Type I error but increases the risk of a Type II error (failing to reject a false null hypothesis). It’s important to consider the impact of these errors on the results of the study and the practical implications in the field of research.
Factors Influencing the Choice of Alpha
- Study Context: In some fields, such as medicine or safety-critical systems, researchers prefer a lower alpha to minimize false positives, even at the expense of higher Type II error rates.
- Sample Size: The larger the sample size, the more sensitive the test becomes, allowing for a higher alpha without compromising the power of the test.
- Consequence of Errors: If a Type I error has more severe consequences (e.g., wrongly approving a drug), then a lower alpha should be chosen. If a Type II error is more critical (e.g., failing to detect a disease), a higher alpha may be preferable.
Choosing an Appropriate Alpha Value
- Start with a default alpha of 0.05 for most common studies.
- If the research has high stakes (e.g., medical trials), consider using a stricter alpha, such as 0.01.
- For exploratory studies or when the cost of Type II errors is high, you might increase alpha to 0.10.
Note: Adjusting alpha should always be justified based on the nature of the study and the potential impact of errors. It’s essential to balance the risk of Type I and Type II errors to draw meaningful and reliable conclusions.
Common Alpha Levels
Alpha Level | Common Use Case |
---|---|
0.05 | Standard threshold for most research studies |
0.01 | High-stakes research (e.g., clinical trials) |
0.10 | Exploratory studies or when Type II errors are more costly |
Understanding P-value Thresholds: 0.05, 0.01, and Beyond
The p-value is a statistical measure used to determine the strength of evidence against the null hypothesis in hypothesis testing. The threshold or significance level (denoted as α) determines how small a p-value must be for the results to be considered statistically significant. Common thresholds include 0.05, 0.01, and in some cases, even stricter values. The choice of threshold depends on the research context, the potential consequences of making errors, and the desired level of confidence in the results.
In hypothesis testing, a p-value less than the chosen threshold suggests that the null hypothesis should be rejected, while a p-value greater than the threshold implies that the null hypothesis is not rejected. However, interpreting the p-value requires careful consideration of its magnitude and the context of the study. Below is an overview of commonly used p-value thresholds.
Common P-value Thresholds
- 0.05: The most widely used threshold. If the p-value is less than 0.05, the result is considered statistically significant, meaning there is strong evidence to reject the null hypothesis.
- 0.01: A more stringent threshold, often used in cases where the consequences of a Type I error (false positive) are more severe. It provides stronger evidence against the null hypothesis.
- 0.001: An even more conservative threshold, typically used in high-stakes or high-precision fields like medical research, where minimizing false positives is critical.
Factors Influencing the Choice of Threshold
- Risk Tolerance: Higher thresholds like 0.05 may be acceptable in exploratory studies, while lower thresholds like 0.01 may be necessary in more critical or definitive research.
- Sample Size: Larger sample sizes can detect smaller effects, making lower thresholds more feasible in ensuring robust findings.
- Context of the Research: In fields such as pharmaceuticals, a stricter threshold might be required to ensure safety and avoid harmful conclusions.
Threshold Comparison Table
Threshold | Common Usage | Risk of Type I Error |
---|---|---|
0.05 | General scientific studies | Moderate |
0.01 | Studies requiring stronger evidence | Low |
0.001 | High-risk or critical research (e.g., medicine) | Very Low |
When deciding on a p-value threshold, researchers must balance the desire for significance with the potential cost of errors. It is crucial to recognize that a lower threshold reduces the chance of a false positive but increases the chance of a false negative.
Limitations of the P-value and Alternative Methods for Statistical Inference
The P-value is commonly used for hypothesis testing, providing a measure of the strength of evidence against the null hypothesis. However, it has several limitations that can lead to misleading conclusions. One primary issue is its dependence on sample size, which can result in small P-values even when the effect is trivial. Additionally, the P-value does not provide the probability of the hypothesis being true, but rather the probability of observing data at least as extreme as the current data, assuming the null hypothesis is true.
Another significant limitation is the threshold-based decision-making, where a P-value below a fixed cutoff (e.g., 0.05) is often misinterpreted as evidence for a real effect. This binary approach disregards the magnitude of the effect, leading to potential overconfidence in findings. To address these concerns, statisticians have developed alternative methods that offer more nuanced approaches to inference.
Alternative Approaches
- Confidence Intervals (CI): Provides a range of values that are likely to contain the true parameter, offering more information than a single P-value.
- Bayesian Inference: Incorporates prior knowledge and updates it with new data, offering a probabilistic framework for hypothesis testing.
- Effect Size: Focuses on the magnitude of the observed effect, independent of sample size, to assess the practical significance of results.
Comparison with P-value
Method | Advantages | Limitations |
---|---|---|
Confidence Interval | Provides a range of possible values for the parameter. | May still be influenced by sample size and variability. |
Bayesian Inference | Incorporates prior information and updates beliefs based on data. | Requires selection of prior distributions, which can be subjective. |
Effect Size | Highlights the practical significance of results. | Does not provide a formal test of hypothesis. |
"P-value does not measure the magnitude of an effect, nor does it provide direct evidence for a hypothesis." - Statistical Consensus