Data Annotation Jobs Work From Home
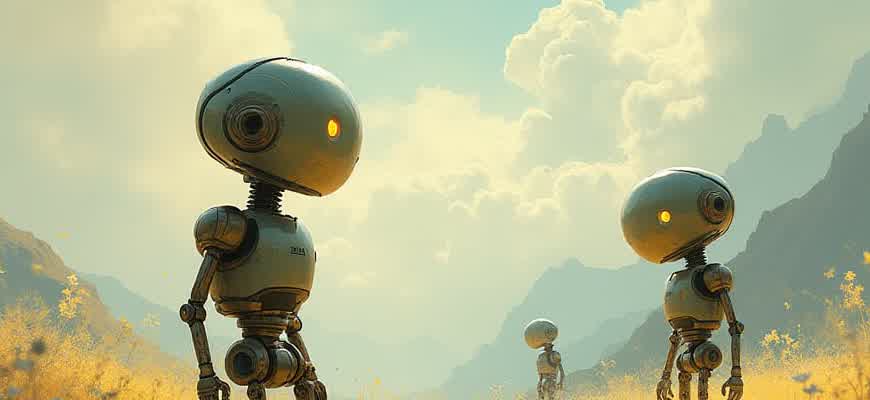
Data labeling is a critical task in the realm of artificial intelligence (AI) and machine learning (ML). Professionals working in this field help improve algorithms by labeling and tagging data accurately. This type of job is particularly well-suited for remote work due to its reliance on digital platforms and tools. Below are some key aspects of data labeling jobs that make them appealing to remote workers:
- Flexible working hours allow individuals to manage their schedules.
- Opportunities to work with leading AI and tech companies.
- Minimal equipment requirements; only a computer and internet connection are necessary.
Job Requirements
- Strong attention to detail.
- Ability to follow specific guidelines and instructions.
- Familiarity with annotation tools such as Amazon Mechanical Turk or Labelbox.
Data annotation is an essential part of machine learning workflows. It's a way to help algorithms understand real-world inputs by labeling images, text, or audio.
In terms of compensation, remote data annotation jobs can vary greatly depending on the company, the complexity of the task, and the number of projects available. Below is a general comparison of common pay rates:
Job Type | Hourly Rate | Task Type |
---|---|---|
Basic Data Labeling | $10 - $15 | Image and text tagging |
Advanced Annotation | $20 - $30 | Complex datasets, AI training |
How to Begin with Remote Data Annotation Jobs
Data annotation jobs are becoming an increasingly popular way to earn money from home. These roles typically involve labeling data for training machine learning models, which can include tasks like image tagging, transcription, and sentiment analysis. As the demand for machine learning and AI grows, so does the need for precise and consistent data annotation.
For individuals interested in starting remote data annotation work, there are several important steps to follow. It's essential to familiarize yourself with the tools and platforms used for annotation, along with understanding the different types of projects you may encounter. Below is a guide on how to begin your career in this field.
Steps to Get Started
- Research Job Platforms: Look for websites that connect freelancers with data annotation projects. Some popular platforms include Appen, Lionbridge, and Clickworker.
- Understand the Requirements: Different annotation tasks require varying skill levels. Some may need specific technical knowledge, while others can be completed with minimal training.
- Prepare Your Workspace: Set up a dedicated work area with reliable internet access and the necessary tools, such as a computer with adequate specifications.
- Complete Training: Many platforms offer introductory courses or tests to help you get familiar with the annotation process. Take these seriously to enhance your chances of being hired.
- Start with Simple Tasks: Begin with basic annotation tasks to build your experience and increase your understanding of the job.
Key Skills to Develop
Before diving into data annotation work, it’s useful to develop specific skills that can improve your efficiency and accuracy.
- Attention to Detail: Data annotation requires precision. Small mistakes can lead to incorrect model training.
- Basic Computer Skills: Familiarity with spreadsheet software, web browsers, and annotation tools is crucial.
- Time Management: Many annotation jobs are paid per task or per hour. Being able to work efficiently is key to maximizing your earnings.
“Accurate and high-quality data annotations are essential for AI systems to perform well. Without this, machine learning models could fail to make correct predictions.”
Expected Earnings and Opportunities
The amount you can earn from data annotation jobs depends on factors like the complexity of the tasks, your experience level, and the platform you work with. Some platforms pay per task completed, while others offer hourly rates.
Platform | Task Type | Pay Range |
---|---|---|
Appen | Image annotation, Transcription | $5 - $20 per hour |
Lionbridge | Text and image annotation | $10 - $25 per hour |
Clickworker | Microtasks, image categorization | $3 - $10 per hour |
Tools and Software You'll Need for Data Annotation Work
Data annotation tasks require a set of specialized tools to streamline the process and ensure high-quality, accurate results. Depending on the type of data you're working with–be it images, videos, or text–the software and platforms you choose must be equipped with specific functionalities. Some tools are designed for manual tagging, while others can automate parts of the process, allowing you to work more efficiently.
In addition to basic hardware requirements such as a reliable computer and internet connection, you will need various tools to manage and label data. These tools can range from open-source software to enterprise-grade solutions, each offering unique features to suit different project needs. Below, we'll outline some of the most essential tools for data annotation.
Essential Data Annotation Tools
- Labelbox: A powerful platform for image, video, and text annotation. It allows you to collaborate with teams and offers AI-assisted labeling.
- VGG Image Annotator (VIA): A free, open-source tool for image annotation that works directly in your browser, ideal for smaller projects.
- Prodi.gy: A machine learning-powered annotation tool for text, image, and video. It helps speed up annotation with active learning.
- RectLabel: A macOS-based tool for image annotation, particularly for bounding box creation in object detection tasks.
- Amazon SageMaker Ground Truth: A fully managed data labeling service that supports text, image, and video annotation with human feedback integration.
Software for Workflow Management
- Jira: A popular project management tool that helps track the progress of annotation tasks, assign responsibilities, and manage deadlines.
- Asana: Useful for organizing tasks, setting milestones, and ensuring that the annotation process runs smoothly across teams.
- Trello: A simple tool for task tracking, allowing you to set up boards and lists for different stages of the annotation process.
Important Features to Look For
Feature | Importance |
---|---|
Collaboration Tools | Allows multiple annotators to work on a project simultaneously and ensures data consistency. |
Data Export Options | Ensures that the labeled data can be exported in the desired format, such as JSON, XML, or CSV. |
AI Assistance | Speeds up the annotation process by suggesting labels based on machine learning models, reducing manual effort. |
Integration Capabilities | Facilitates seamless integration with other tools in your workflow, such as cloud storage or version control systems. |
Note: The choice of annotation tool depends largely on the project’s scale, the complexity of the data, and your team's collaboration needs. Make sure to evaluate the software's features before committing to one, as it can significantly impact the efficiency of your workflow.
Understanding Different Types of Data Labeling: Text, Image, and Video
Data labeling plays a critical role in training machine learning models. The process involves tagging or annotating raw data to provide context that enables AI algorithms to learn patterns. There are several types of data annotation depending on the nature of the data, including text, image, and video annotations. Each of these has its own unique set of challenges and requires specialized tools and expertise.
This article explores the three primary types of data annotation: text, image, and video, highlighting their specific applications, techniques, and importance in the machine learning ecosystem.
Text Annotation
Text annotation involves labeling textual data to help machines understand language, sentiment, and context. It is essential for various applications like natural language processing (NLP) and sentiment analysis. Common tasks in text annotation include categorizing, tagging, and extracting specific information from the text.
- Named Entity Recognition (NER): Identifying entities such as names, locations, dates, etc.
- Sentiment Analysis: Categorizing the emotion or opinion expressed in the text.
- Text Classification: Sorting text into predefined categories based on content.
Image Annotation
Image annotation involves labeling objects or features in visual data, which is crucial for computer vision tasks. This type of annotation helps algorithms to recognize, interpret, and categorize visual information. Annotating images can include tasks like identifying objects, outlining boundaries, or labeling specific features.
- Bounding Boxes: Drawing rectangles around objects for object detection.
- Semantic Segmentation: Classifying each pixel in an image for detailed analysis.
- Keypoint Annotation: Marking specific points on objects for precise location tracking.
Video Annotation
Video annotation extends the principles of image annotation to dynamic content. It is crucial for tasks like activity recognition, object tracking, and facial expression detection. Video annotation often involves tagging each frame or section of the video to provide temporal context and enhance model training.
Task Type | Description |
---|---|
Action Labeling | Annotating actions or behaviors in video content (e.g., walking, jumping, etc.) |
Object Tracking | Labeling objects across frames to track their movement or changes over time. |
Event Detection | Identifying and labeling significant events or transitions within video content. |
“Data annotation is the backbone of machine learning, enabling algorithms to accurately interpret and respond to real-world data.”
How to Find Legitimate Data Annotation Job Offers Online
Finding legitimate online data annotation opportunities can be challenging, but with the right approach, it’s possible to secure a trustworthy and well-paid position. The rise of remote work has opened up many possibilities in this field, but it has also led to an increase in scams. It’s essential to learn how to differentiate between credible job offers and those that may be too good to be true.
To ensure you are applying to legitimate data annotation jobs, follow a structured process. You’ll need to verify the reputation of companies, use trusted platforms, and avoid jobs that seem suspicious. Here are some useful tips on how to spot credible offers and avoid falling into common traps.
Steps to Identify Genuine Data Annotation Jobs
- Research the Company: Always check the company’s website and search for reviews. Trustworthy businesses typically have a professional online presence and positive user feedback.
- Use Reliable Job Platforms: Look for job listings on well-established platforms like LinkedIn, Glassdoor, or Indeed. These websites have strict vetting processes that help filter out fraudulent job postings.
- Avoid Upfront Fees: Legitimate data annotation companies will never ask for payment before offering you work. If a job listing asks for a fee to apply or access training materials, it’s likely a scam.
Red Flags to Watch Out For
“If it seems too good to be true, it probably is.” Watch out for high-paying jobs that require minimal effort or offer unrealistic salary promises.
- Unrealistic Salary Offers: Be cautious of job offers that promise an unusually high income with little work involved. Data annotation typically offers modest hourly rates, especially for entry-level positions.
- Vague Job Descriptions: Legitimate job offers will clearly explain the responsibilities, expected hours, and payment structure. Avoid listings that provide minimal information or are overly vague.
- Suspicious Contact Methods: Verify that the company provides professional contact information, such as a company email and phone number. Be wary of jobs that only communicate via messaging apps like WhatsApp.
Trusted Websites for Data Annotation Jobs
Website | Description |
---|---|
Appen | A reputable platform for freelance data annotation jobs with a wide variety of tasks and global opportunities. |
Lionbridge | Offers remote work opportunities for data annotation, translation, and content moderation across various industries. |
Clickworker | Provides a variety of microtasks, including data annotation, with flexible working hours and reliable pay. |
What Skills Are Essential for Data Annotation Jobs?
Data annotation roles, especially in the remote work environment, require a specific set of skills to ensure the accuracy and efficiency of the task. These jobs often involve labeling or categorizing data, which can range from images and videos to text and audio. For anyone to thrive in this field, a few core competencies are necessary to meet the demands of the job and to deliver quality results consistently.
Though the work can be repetitive, a high level of attention to detail and a solid understanding of the data involved are crucial. Successful data annotators need a mix of technical and soft skills to effectively manage tasks and collaborate with teams, often remotely. Below are the primary skills required for success in this field.
Key Skills for Success in Data Annotation Roles
- Attention to Detail: Accurate data labeling is crucial, and even small errors can lead to significant issues in machine learning models.
- Familiarity with Annotation Tools: Proficiency with specialized software and tools used for data annotation (e.g., CVAT, Labelbox, or Prodigy) is essential.
- Time Management: Data annotation tasks often come with deadlines. The ability to manage time effectively ensures the completion of projects on schedule.
- Communication Skills: Clear communication is necessary, especially in a remote setup, for asking questions, providing feedback, or clarifying project requirements.
- Understanding of the Data Domain: Familiarity with the specific type of data you're annotating (e.g., medical images, text, etc.) can improve the accuracy and quality of annotations.
Additional Technical and Analytical Competencies
- Analytical Thinking: Being able to recognize patterns and anomalies in data helps annotators understand the context and apply the correct labels.
- Adaptability to New Technologies: The field of data annotation evolves quickly, requiring the ability to learn new tools or techniques as they emerge.
- Basic Knowledge of Machine Learning Concepts: Understanding how annotated data is used in model training can help improve the annotation process and outcome.
Remember, data annotation is not just about labeling data, but ensuring the labels are meaningful and useful for the intended machine learning application.
Commonly Used Annotation Tools
Tool | Type of Annotation |
---|---|
CVAT | Image, Video, and Object Detection |
Labelbox | Text, Image, Video |
Prodigy | Text Annotation (e.g., Named Entity Recognition) |
How to Stay Organized and Efficient While Working from Home
Remote data annotation jobs offer flexibility, but they also present unique challenges when it comes to managing your time effectively. Without the structure of a traditional office environment, it's easy to lose track of tasks or let distractions take over. However, with a few smart strategies, you can maintain focus and productivity throughout your workday.
In order to balance productivity and personal time, it’s important to implement a few key habits. Setting clear boundaries, using tools for task management, and maintaining a structured routine will help you stay on track and meet your deadlines. Here are some practical methods to manage your time while working from home.
Tips for Effective Time Management
- Set Clear Work Hours: Define a specific start and end time each day. This helps establish a routine and prevents work from bleeding into personal time.
- Prioritize Tasks: Organize tasks based on deadlines and importance. Focus on completing high-priority work first.
- Avoid Multitasking: Stick to one task at a time. Multitasking can reduce efficiency and increase errors in annotation tasks.
Tools and Techniques to Stay Productive
- Use Time-Tracking Apps: Apps like Toggl or RescueTime help monitor how much time you spend on each task, providing insight into your work habits.
- Create a Distraction-Free Workspace: Set up a dedicated area for work to minimize distractions from home life.
- Take Regular Breaks: Use techniques like the Pomodoro method (work for 25 minutes, then take a 5-minute break) to keep your mind fresh.
Key Tools to Improve Focus
Tool | Purpose |
---|---|
Todoist | Task management and organization |
Focus@Will | Music designed to boost concentration |
Forest | Helps stay focused by planting virtual trees |
"Managing time effectively is not just about getting work done–it's about ensuring that your workday stays sustainable and you don't burn out."
Common Challenges in Data Annotation Jobs and How to Overcome Them
Data annotation jobs offer flexible work-from-home opportunities, but they come with their own set of challenges that can impact efficiency and quality. One of the most common issues is ensuring high accuracy in labeling data, especially when dealing with large volumes of unstructured information. This challenge can be intensified by the lack of clear guidelines or ambiguous project requirements.
Another difficulty is managing repetitive tasks that can lead to fatigue and reduced concentration over time. Annotation jobs often require long hours of repetitive work, which can result in mistakes if not properly managed. To overcome these challenges, workers need to implement strategies that improve focus and maintain quality standards throughout the process.
Key Challenges in Data Annotation Jobs
- Ambiguity in Instructions: Often, project guidelines are unclear, leading to inconsistencies in the data labeling process.
- High Workload: Handling large datasets can be overwhelming, especially when dealing with complex data types.
- Fatigue: Repetitive tasks can cause mental exhaustion, leading to mistakes and lower productivity.
Strategies to Overcome These Challenges
- Clarify Guidelines: Ensure that instructions are clear and concise. If necessary, seek clarification from project managers before beginning the task.
- Time Management: Break down the task into smaller, manageable parts and take regular breaks to maintain focus.
- Use Tools for Quality Control: Employ software tools designed to assist with annotations, providing automatic suggestions or highlights to improve accuracy.
Tip: Consistently review the completed annotations for accuracy, and if possible, collaborate with other annotators for cross-validation.
How to Tackle Workload and Fatigue
Approach | Description |
---|---|
Breaks | Incorporate regular short breaks into your schedule to avoid burnout and maintain focus. |
Task Rotation | Switch between different types of annotation tasks to prevent monotony and keep your mind engaged. |
Use Ergonomic Tools | Invest in a comfortable chair, desk setup, and keyboard to reduce physical strain and improve productivity. |