What Is Simple Sampling Method
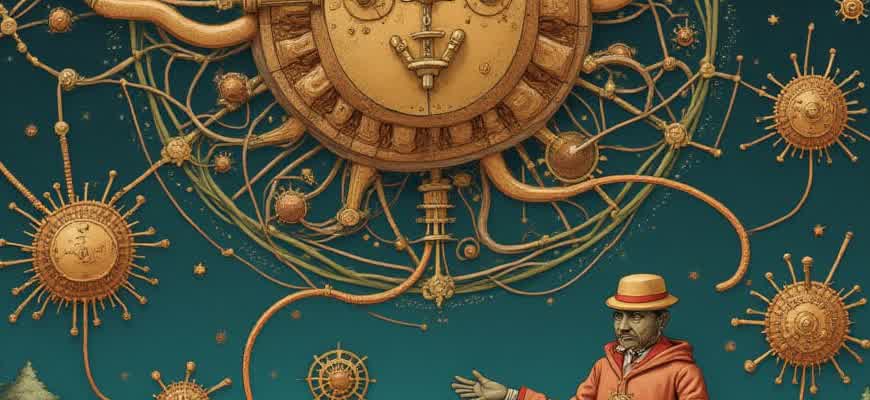
Simple sampling is a basic technique used in statistical research to select a subset from a larger population. This method is known for its ease and efficiency, as every individual in the population has an equal chance of being selected. The process involves choosing a random sample without any bias, making it a popular choice for various studies and surveys.
The process of simple sampling can be broken down into the following steps:
- Define the population from which the sample will be drawn.
- Assign a number to each individual or unit in the population.
- Randomly select the required number of units or individuals from the population.
Note: It is crucial that the selection process is random to maintain the objectivity of the sample. This reduces any potential bias and ensures that the results are representative of the population.
There are several methods to achieve randomness in simple sampling:
- Lottery Method: Numbers are written on slips of paper, placed in a container, and drawn randomly.
- Random Number Table: Numbers are selected using a table that contains randomly generated numbers.
- Computer Generated Random Numbers: A software or tool generates random numbers to select participants.
By using these techniques, researchers can ensure that the sample they choose reflects the diversity and characteristics of the entire population.
Understanding the Basics of Simple Sampling
Simple sampling is one of the most straightforward methods for selecting a subset of individuals from a larger population. This approach is widely used in statistics because it is easy to implement and ensures that every individual in the population has an equal chance of being included in the sample. The key objective is to gather data from a small, representative group, which can then be generalized to the entire population.
The process begins by defining the population clearly. Once the population is identified, every individual or item is given an equal opportunity to be selected. This randomness is what makes simple sampling effective in avoiding bias, ensuring that the sample is as representative as possible of the broader group.
Steps Involved in Simple Sampling
- Define the population and sampling frame.
- Determine the sample size based on the study’s requirements.
- Use a random method (e.g., lottery, random number generator) to select the sample.
- Collect data from the selected sample.
Key Considerations
The main advantage of simple sampling is its simplicity and low cost, especially when the population is small and manageable. However, it may be less effective for larger populations where a more stratified or complex sampling method might be required to ensure diversity in the sample.
Example of Simple Sampling Process
Step | Action |
---|---|
1 | Identify the population: all students in a school. |
2 | List all individuals: create a roster of students. |
3 | Select a random sample: choose students randomly from the list. |
4 | Collect data: survey the selected students. |
Key Steps in Implementing Simple Sampling
Implementing simple sampling is a straightforward process that ensures every element in the population has an equal chance of being selected. This method is highly effective when dealing with large populations, and it simplifies the sampling procedure. The key steps involve defining the population, creating a sampling frame, and selecting the sample using a random method.
Each of these steps must be followed carefully to maintain the integrity and randomness of the sample. If done correctly, simple sampling can yield a representative sample that mirrors the population’s characteristics, allowing for valid inferences to be drawn.
Steps to Implement Simple Sampling
- Define the Population: Clearly identify the population that you want to study. The population must be well-defined and relevant to the research question.
- Create a Sampling Frame: Develop a comprehensive list of all members within the defined population. This list serves as the sampling frame from which you will randomly select your sample.
- Random Selection: Use a random process (such as a random number generator or drawing lots) to choose the sample from the sampling frame. Every element must have an equal chance of selection.
- Determine the Sample Size: Decide how many individuals or units to select. The sample size depends on factors such as the desired level of precision and the overall size of the population.
Simple sampling ensures that every individual in the population has an equal probability of being selected, which minimizes selection bias and enhances the generalizability of results.
Example of Simple Sampling Process
Step | Description |
---|---|
1. Define Population | Identify all individuals that fall under your study criteria. |
2. Create Sampling Frame | Generate a list of all population members. |
3. Random Selection | Randomly pick individuals from the list using a random method. |
4. Determine Sample Size | Choose how many individuals to include in the sample. |
Common Mistakes in Simple Sampling and How to Avoid Them
In the process of simple sampling, researchers aim to create a sample that represents the entire population. However, several mistakes can undermine the integrity of the sample and lead to skewed results. Understanding these pitfalls is essential for ensuring the accuracy of any findings drawn from the sample.
One of the most common errors in simple sampling is not properly identifying the sampling frame. This leads to a sample that may not be representative of the larger population, skewing results and reducing the reliability of conclusions.
Common Mistakes
- Inaccurate Sampling Frame: A sampling frame that does not fully encompass the target population can lead to biased samples.
- Failure to Randomize: Not ensuring each member of the population has an equal chance of being selected can create a non-random sample.
- Sample Size Issues: Choosing a sample that is too small or too large can affect the reliability of the data.
- Excluding Specific Groups: Deliberately or accidentally excluding certain segments of the population reduces the representativeness of the sample.
How to Avoid These Mistakes
- Ensure Complete Sampling Frame: Carefully define and use a sampling frame that accurately reflects the entire population.
- Implement True Randomization: Use a random number generator or other unbiased methods to select samples.
- Determine an Adequate Sample Size: Calculate an appropriate sample size based on statistical principles to ensure data reliability.
- Include All Relevant Groups: Take steps to ensure every group within the population is represented in the sample, avoiding over-representation or under-representation.
Important: A well-executed simple sampling method can lead to valid and reliable results. Avoiding these common errors is key to ensuring that the findings are both accurate and generalizable to the entire population.
Example of Proper vs. Improper Sampling
Improper Sampling | Proper Sampling |
---|---|
Using a list of phone numbers from a single area code | Using a national phone directory with random selection |
Selecting a sample based on convenience (e.g., survey a nearby group of people) | Ensuring every individual has an equal chance of being selected from the population |
When to Use Simple Sampling in Research Projects
Simple sampling is an appropriate method to use when researchers need a straightforward, unbiased way to select a sample from a larger population. This technique works well in situations where the entire population is relatively homogeneous, making it easier to generalize findings. It is particularly useful when there is no prior knowledge of subgroups within the population, and the goal is to ensure every individual has an equal chance of being selected.
Additionally, simple sampling is effective when the researcher has limited time, resources, or access to the full population. This method avoids the complexity of stratified or cluster sampling, making it ideal for quick studies with less stringent requirements for accuracy or detail in subgroup analysis.
When to Apply Simple Sampling
- Homogeneous Population: When the population is similar across various characteristics, simple sampling allows for unbiased and straightforward representation.
- Limited Resources: If the researcher is working with a small budget or time constraints, simple sampling provides a cost-effective and quick solution.
- Generalization of Findings: When the research aims to make broad generalizations about the entire population, simple sampling ensures every member has an equal probability of being selected.
Key Considerations
Simple sampling works best when there is no prior information about the subgroups within the population. If such groups exist and may influence the research outcomes, other methods like stratified or cluster sampling might be more suitable.
- Ensuring Randomness: The process of selection must be random to avoid bias. Every individual should have an equal chance of being included.
- Sample Size: A larger sample size is generally required to ensure the findings are statistically significant and representative of the population.
Example of Simple Sampling in Practice
Scenario | Sampling Method | Reason |
---|---|---|
University Students | Simple Random Sampling | All students are considered part of a homogeneous group with no known subgroups influencing the outcome. |
Consumer Product Testing | Simple Random Sampling | The target market is large and diverse, and random sampling allows for broad generalizations. |
Benefits of Using Simple Sampling for Data Collection
Simple sampling is a widely used technique in research due to its ease of application and clear benefits. By selecting a random subset from a larger population, it ensures that every individual has an equal chance of being included, reducing bias in the data collection process. This makes the method particularly valuable when the goal is to gather unbiased data from a representative segment of the population.
Using simple sampling provides several advantages in data collection, making it a go-to approach in various fields. The simplicity of the technique, coupled with its cost-effectiveness, allows researchers to quickly gather data without requiring extensive resources or complex procedures. Below are the key benefits of adopting this method.
Key Benefits
- Cost-Effective: Simple sampling does not require sophisticated tools or complicated procedures, making it a low-cost approach for data collection.
- Time-Efficient: Since the method involves random selection, researchers can easily and quickly select participants without the need for detailed stratification or categorization.
- Reduces Bias: The random nature of simple sampling ensures that all individuals have an equal chance of being selected, reducing the possibility of biased outcomes.
"Simple sampling is particularly effective in cases where the population is large and researchers need a straightforward way to gather a representative sample without incurring significant costs."
Comparison with Other Sampling Methods
Method | Cost | Time | Bias Reduction |
---|---|---|---|
Simple Sampling | Low | Fast | High |
Stratified Sampling | Moderate | Moderate | Moderate |
Systematic Sampling | Low | Fast | Moderate |
"Compared to other methods, simple sampling strikes a balance between cost, speed, and minimizing bias, making it ideal for many research projects."
Tools and Techniques for Conducting Simple Sampling
Simple sampling techniques rely on easy-to-use tools and strategies to ensure that every member of a population has an equal chance of being selected. The methods employed should be both efficient and random, avoiding bias and guaranteeing the representativeness of the sample. The tools used for simple sampling can range from manual methods to more advanced technologies, depending on the size of the population and the complexity of the process.
Among the most common techniques are random number generators and drawing lots. These tools can be easily applied in both small-scale and large-scale sampling projects. Additionally, statistical software offers more sophisticated options, ensuring precision when working with large datasets.
Common Techniques
- Random Number Generation: Software or manual methods that assign numbers to each individual in a population and randomly select samples.
- Drawing Lots: A physical method where individuals' names or identifiers are placed into a container and drawn randomly.
- Use of a Random Sampling Table: A pre-generated list of random numbers or sequences that can be used to choose sample members.
Tools for Simple Sampling
Tool | Application |
---|---|
Random Number Generator | Used for generating random selections from a list of items. |
Random Sampling Tables | Provides pre-made sets of random numbers for sample selection. |
Drawing Lots | A physical method often used in small groups or informal settings. |
Important: When using any of these tools, it is essential to ensure that the sample is truly random and that no systematic bias influences the selection process.